Financial News
Snorkel Launches Advanced Data-Centric AI Capabilities for Evaluation and Fine Tuning of LLM and RAG Systems
New features reduce the time, cost and complexity of data preparation across predictive and generative AI development life cycles
Snorkel AI announced new capabilities in Snorkel Flow, the AI data development platform, to accelerate the specialization of AI/ML models in the enterprise. These capabilities include GenAI evaluation tools for use-case-specific benchmarks, streamlined LLM fine-tuning workflows and advanced named entity recognition (NER) for PDFs—all of which strengthen Snorkel Flow’s position as the only platform capable of supporting the entire AI data development lifecycle.
This press release features multimedia. View the full release here: https://www.businesswire.com/news/home/20241009219472/en/
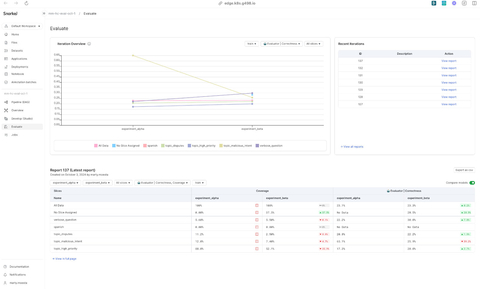
(Graphic: Business Wire)
“AI-ready data” is critical for enterprises to build specialized AI models leveraging proprietary, unstructured data for real-world use cases. According to Gartner, “AI-ready data means that your data must be representative of the use case, of every pattern, error, outlier and unexpected emergence that is needed to train or run the AI model for the specific use. Data readiness for AI is not something you can build once and for all, nor that you can build ahead of time for all your data.”
Snorkel Flow’s latest release equips enterprises with a robust platform to implement and scale AI data development practices—and accelerate production delivery of highly accurate, specialized AI models.
Key new features include:
- LLM evaluation tools: You can now customize evaluation for domain specific use cases, get fine-grained insight into error modes and seamlessly step into data development to fix errors. Snorkel Flow lets you add custom acceptance criteria and validate each with out-of-the-box or custom evaluators to assess LLM performance for specific enterprise use cases, delivering the insights and confidence needed to push specialized models to production. Further, the introduction of data slicing uncovers areas where additional training data is needed.
- RAG tuning workflows: Improve retrieval accuracy with advanced chunking, fine-tuning embedding models and document metadata extraction to support hybrid semantic-keyword search. These workflows significantly reduce the development time needed to improve the quality of AI copilots and assistants whose responses must be grounded by enterprise policies and data.
- Named Entity Recognition (NER) for PDFs: Extract information from PDFs easier and faster than ever by simply clicking on words, drawing bounding boxes, specifying patterns and prompting foundation models. The flexibility to easily capture information based on visual structure and spatial relationships simplifies, accelerates and improves the accuracy of NER models.
- Simplified annotation and feedback: Enjoy a faster, streamlined workflow that makes it easy for subject matter experts to annotate data with simplified interfaces for multi-criteria evaluation and free text response collection—allowing them to annotate data and provide feedback at the same time.
- Sequence tagging analysis tools: Visually zero in on model prediction errors in sequence tagging workflows using the new Spotlight Mode. Additionally, class-level metrics (e.g., F1, precision) are now available in model iteration graphs and analysis tables to provide more granular insight into performance.
- User experience enhancements: Facilitate collaboration between subject matter experts (SMEs) and data scientists by standardizing on a platform that brings together participants from across the organization. A wide range of UX improvements make this experience far smoother, by making navigation, workflows and data labeling easier and more intuitive.
- AI ecosystem integration: Integrate seamlessly with leading AI development platforms by taking advantage of deeper integration with Databricks Data Intelligence Platform and Amazon SageMaker to fine tune and deploy specialized models, as well as expanded support for LLM prompting with popular foundation models such as OpenAI ChatGPT, Google Gemini and Meta Llama to accelerate data labeling and evaluation.
“AI is at the top of every enterprise leader’s priority list, but the deep work needed for consistent, repeatable AI development is daunting, costly and manual,” said Alex Ratner, CEO and co-founder of Snorkel AI. “Data underpins successful adoption of AI in large enterprises, which is why these updates to our AI data development platform are so important. They’re fundamental to helping enterprises accelerate and optimize the delivery of AI solutions.”
Resources:
- See new Snorkel Flow features in action
- Follow exclusive insights from SnorkelCon, Snorkel’s sold out user conference
- Discover innovative data centric AI research done by Snorkel
- Find case studies, eBooks and more in Snorkel’s Resource Center
- Join a live workshop to get hands on with Snorkel Flow
- Watch a demo and participate in live Q&A with our ML experts
About Snorkel AI
Snorkel AI makes enterprise AI data development programmatic. With the world’s first AI data development platform, Snorkel Flow, companies can use their proprietary data to customize AI models for their business needs faster and more easily than ever before. Launched out of the Stanford AI Lab, Snorkel is used in production by Fortune 500 companies including BNY, Wayfair and Chubb, as well as across the federal government, including the US Air Force. Visit snorkel.ai, and follow us on LinkedIn and @SnorkelAI on X, for more information.
View source version on businesswire.com: https://www.businesswire.com/news/home/20241009219472/en/
Contacts
Media: snorkel@inkhouse.com
Stock quotes supplied by Barchart
Quotes delayed at least 20 minutes.
By accessing this page, you agree to the following
Privacy Policy and Terms and Conditions.